From Backtesting to Arbitrage: Practical Applications of AI in the World of Retail Trading
In this article, the industry leaders delve into the transformative role of AI in trading.
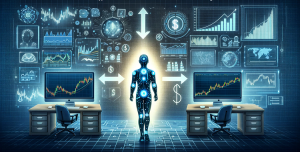
Shawn Carpenter, the Chairman and CEO of StockAlarm, analogizes AI in trading to self-driving technology. Josh Kincaid, CMO at ToroAlerts, critically examines AI’s evolving role. Anthony Cammarano, a seasoned expert in cybersecurity, discusses AI’s implications for data security within trading strategies. Bernard Marr, a generative AI expert, delves into AI’s revolutionary impact on the trading industry. Giuseppe Sette, President of Toggle AI, discusses AI’s unmatched data analysis capabilities. Sean Kozak, developer of NeuroStreet, highlights practical AI applications in algorithmic trading, and Brian Prince, CEO of TopAITools.com, offers a nuanced perspective on AI in the crypto trading realm.
AI trading, the integration of artificial intelligence into the trading process, is shaping up to be a game-changer, much like the internet, electricity, and the steam engine were in their times. While it’s hard to predict exactly where it’s headed, it’s clear that AI’s impact could be felt sooner than we think.
Overall, AI trading leverages algorithms and machine learning to process large volumes of data, identify market patterns and trends, minimize human error, and uncover opportunities that might be overlooked by traditional methods.
Overview of AI Trading
AI trading executes trades using computer algorithms that analyze data like historical price movements and economic indicators to inform trading decisions.
Over time, AI trading has advanced, incorporating more complex machine learning algorithms. These developments have enabled traders to make more informed decisions and, for some, automate their trading strategies to capitalize on market opportunities around the clock.
AI trading tools also offer an edge by helping investors overcome common biases and emotional decisions. They can execute trades quickly, seizing opportunities that might otherwise be missed, ultimately leading to better outcomes and higher returns.
Additionally, these tools provide real-time updates and alerts, allowing investors to adjust their strategies promptly in response to market movements.
However, it’s essential to understand that AI tools supplement rather than replace human judgment and expertise.
Practical Use Cases of AI in Retail Trading
AI trading applications represent a sophisticated blend of artificial intelligence and machine learning, offering a range of benefits to retail investors. Here is an overview of real-world applications for AI in retail trading, with some already in operation while others have the potential to emerge.
AI trading bots
At the core of AI trading are bots, specialized programs that perform tasks like trend analysis, market movement prediction, and identifying investment opportunities, tailored to user preferences. These bots are essential for navigating markets like cryptocurrency and forex, becoming vital assets for financial entities.
AI trading bots excel by leveraging algorithms to spot trends and pinpoint profitable trading opportunities. Their ability to autonomously execute trades makes them particularly advantageous for OTC trading.
Quantitative Analysis
A key technique in quantitative analysis is regression analysis, which examines the relationship between multiple variables to identify trends and correlations. By employing AI, traders can devise strategies to capitalize on the interplay between different market factors or asset correlations, exploiting inefficiencies for profit.
High-Frequency Trading (HFT)
High-frequency trading represents the epitome of speed in AI trading, executing transactions in mere fractions of a second. HFT strategies leverage advanced algorithms and high-speed data transmission to profit from minor price fluctuations, trading large volumes quickly and efficiently.
Arbitrage Strategies
Arbitrage strategies seek to benefit from price discrepancies across different markets or assets. By identifying mispriced assets relative to each other, traders can buy and sell these assets to capture the price difference as profit.
Predictive Models
Machine learning’s primary application in trading is the development of predictive models. These AI models analyze historical market data to forecast future trends, providing traders with insights into potential price movements.
Sentiment Analysis
Sentiment analysis represents another innovative use of AI in retail trading. By analyzing news articles, social media, and other information sources, traders can gauge market sentiment, informing their buy or sell decisions based on the prevailing mood of the market.
Reinforcement Learning
Reinforcement learning allows algorithms to learn from their actions, adjusting strategies based on the outcomes of past trades. This adaptive approach creates dynamic trading models that evolve in response to market changes, optimizing strategies for real-time conditions.
Data Mining
Data mining plays a pivotal role in AI trading by sifting through extensive datasets to detect patterns and market trends. Utilizing algorithms and statistical models, data mining digs into historical market data, uncovering actionable insights that inform trading strategies.
Real-Time Analytics
This process involves analyzing data in real time, employing algorithms and statistical models to detect patterns and trends. The ability to process information on the fly offers AI trading systems a significant edge, enabling rapid response to market changes.
Risk Assessment Models
Risk assessment models are vital for evaluating the potential outcomes of trading decisions. By analyzing historical data, these models estimate the likelihood of various market scenarios.
Stress Testing
Stress testing evaluates a trading strategy’s resilience under various market conditions, revealing potential strategy weaknesses. By simulating different market scenarios, traders can adjust their strategies to mitigate identified risks.
Automated Trading Systems
Automated trading systems execute trades based on predefined rules and criteria, utilizing AI to analyze market data and pinpoint trading opportunities. These systems automate the trading process, reducing the need for constant manual oversight and allowing for efficient market participation.
Backtesting
Backtesting is a critical advantage offered by AI, allowing for the simulation of trading strategies using historical data. This process not only assesses a strategy’s viability but also identifies potential improvements. For instance, AI tools can analyze stocks poised for breakouts, advising on the most effective options strategies based on historical performance.
Benchmarking
Benchmarking complements backtesting by comparing a strategy’s performance against a selected benchmark or market index. This comparison helps traders understand how their strategy fares relative to the market or specific sectors, providing insights into its relative success.
Challenges and Limitations
Despite the advantages, AI trading faces several challenges and limitations:
Market Volatility
AI trading systems, trained on historical data, may struggle to predict unexpected market events or black swan incidents, leading to inaccuracies and financial losses. Furthermore, the widespread use of AI algorithms can amplify market volatility, as simultaneous algorithmic reactions to market signals may exacerbate price fluctuations.
AI Interpretability
The complexity and opacity of AI models pose interpretability challenges, making it difficult for traders to understand the rationale behind algorithmic decisions. This opacity can erode trust in AI systems, complicating informed decision-making. Additionally, AI models are susceptible to data inaccuracies.
Wrap-Up
Pros
AI makes things faster and smarter indeed. Manual trading might not cut it in the future as most trading is done by robots, especially in high-speed and arbitrage trading. High-speed trading is about making lots of trades super fast. Arbitrage involves trading in different markets at the same time to take advantage of price differences.
Not long ago, stock trading happened on big, noisy floors filled with traders. But then computers changed everything, making trading electronic and accessible worldwide. This shift left many traditional traders behind, as they couldn’t keep up with the new digital market.
The appeal of AI trading lies in its potential to make sophisticated trading strategies accessible to newcomers, reducing emotional biases and improving risk management. However, the limitations of AI, including the lack of human intuition and adaptability to unexpected market changes, highlight the importance of a balanced approach to automation in trading.
Cons
Science fiction often makes AI seem like a super-smart force. But really, AI today is just a bunch of algorithms, or steps to solve problems, that range from simple to very complex. Some algorithms are so complicated that even experts find them hard to explain. Others are simple enough for a computer science student to program and win a game of checkers. It’s not about which one is better; they just do different things with different kinds of data.
AI can look at huge amounts of data to find patterns and give useful information. But success in retail trading still needs people to make decisions and handle problems that AI can’t predict. So, despite some scary predictions, the teamwork between humans and AI is still the most likely path forward.
Conclusion
Trading with AI or by hand has its pros and cons. That’s why many big companies use both AI and expert traders. They work together to make more money and reduce risks.
AI algorithms are incredibly quick and accurate. They can handle huge amounts of data in milliseconds and execute trades perfectly. Human traders can’t compete with AI in speed. AI can also read the market and financial news super fast, something humans can’t match.
Robots don’t get tired or emotional. They don’t take breaks, get sick, or make decisions based on feelings like greed or fear. However, AI today doesn’t have the intuition or creativity humans do. They follow their programming, but they’re not great at seeing the big picture. That’s why humans still play a big role in making big investment decisions.
In summary, robots are a big deal in retail trading now, doing more trading than humans. AI has many advantages, like being fast, accurate, and unemotional. But humans still have the upper hand in intuition and creativity. This might change as AI gets better, possibly leading many traders to leave the industry or invest in trading algorithms themselves. One thing’s for sure: the financial world is always evolving.
Industry Insights
Brian Prince, CEO of TopAITools.com and founder of the crypto trading platform XCoins, provides a nuanced perspective on the role of AI in retail trading.
Prince points out, “AI systems lack the inherent bias and emotion of human traders.” However, he also cautions that any bias in AI systems stems from their human developers.
Despite the hype around AI’s performance, Prince is skeptical about its proven superiority over human traders in the long term, stating, “I personally have not seen any instances of this.”
On the accessibility of AI trading tools, Prince observes that many platforms are designed to be user-friendly for retail investors. He mentions Uptrends AI as an example of an intuitive platform that leverages natural language processing to inform investors.
Prince also addresses AI’s role in risk management and predictive analysis, noting its capability to process vast data volumes swiftly. This efficiency can reveal market patterns and risks overlooked by human traders. Nevertheless, he emphasizes, “AI is only a tool,” arguing that while AI can support informed decision-making, it cannot replace the nuanced advice of human financial advisors.
Regarding AI’s impact on market dynamics, Prince notes, “AI tools can enhance market efficiency and liquidity…they can also contribute to increased volatility”.
Sean Kozak, the developer of NeuroStreet, als provided insights into the real-world applications of AI by retail traders. He highlights the use of AI in developing algorithmic and quant systems for trading platforms, which eliminates the need for individual coding and reduces the financial burden of hiring programmers.
Regarding AI-driven strategies among retail traders, Kozak notes the difficulty in pinpointing effective strategies, suggesting that many may not deliver substantial value. “This is a very hard question to answer as most strategies used by retail are not worth their weight in gold.”
Kozak also addresses the perceived impact of AI trading bots and algorithms on market volatility and liquidity, dismissing it as largely overhyped. He argues that significant market movements are primarily driven by institutional actions rather than retail trading algorithms, pointing out the distinction between marketing narratives and the actual influence of trading volume.
“It doesn’t, this is just hype. The only impact on volatility and liquidity comes from large SIZE. And only institutions have this, the rest is just marketing and bull@#$%.”
On regulatory challenges, Kozak points to a broader issue within the industry: a lack of clarity and understanding of AI’s regulatory landscape, even among governing bodies like the SEC.
Giuseppe Sette, the President and co-founder of Toggle AI, shares insights on the integration of AI in trading, highlighting its superior capability to analyze vast datasets which is not feasible for human traders.
He notes, “They can go both ‘deep and wide’…at a scale that is just not feasible for human traders.”
However, Sette clarifies that this does not necessarily set AI in opposition to human traders. Instead, AI serves as a tool that unveils investment opportunities, while human traders contribute a holistic perspective, crucial for identifying the best investment options.
Highlighting the practical success of AI, Sette mentions that “The greatest quant hedge funds and market makers have effectively taken the top spot in terms of risk-adjusted performance 10 to 15 years ago and never let it go”.
Sette also addresses the barriers to AI integration in trading, pointing out the necessity for a blend of AI and investing skills to fully leverage AI capabilities.
Sette further elaborates on AI’s role in risk management, stating it not only expands the horizon of traditional risk management but also helps in predicting future scenarios and identifying emerging macro trends, such as the implications of AI on inflationary pressures due to workforce replacement.
Bernard Marr, a generative AI expert and author, delves into the impact of AI on the trading industry, offering direct insights into how these systems revolutionize market operations.
“AI systems are transforming the trading landscape by offering superior performance and efficiency compared to traditional trading strategies,” Marr explains. He points out, “There have been instances where AI-driven strategies have consistently outperformed human traders,” especially in high-frequency trading where time is critically short.
Marr addresses the challenges of integrating AI into trading, noting the substantial barriers in terms of “technical knowledge and financial resources.” Yet, he also highlights the democratizing potential of cloud computing and AI trading platforms, which “are beginning to lower these barriers for retail traders.”
Retail traders are increasingly relying on AI for a variety of functions, including “algorithmic trading, sentiment analysis from news and social media to gauge market mood, and automated risk management.”
However, Marr does not shy away from discussing the complexities introduced by AI, acknowledging that while it can improve “market efficiency and liquidity,” it might also “lead to increased short-term volatility.” Marr concludes with a forward-looking perspective, emphasizing that “the key to harnessing the power of AI in trading lies in balancing innovation with ethical considerations and regulatory compliance.”
Anthony Cammarano, with over 25 years of experience in cybersecurity and technology, brings a focused perspective on the intersection of AI, data security, and trading strategies.
As the Global VP of Security, Privacy & Strategy at Protegrity, Cammarano highlights, “AI systems have a unique capacity to ingest massive amounts of data and effectively summarize and identify patterns”. This capacity enables AI to excel in both short-term decision-making, leveraging vast datasets in fractions of a second, and in navigating complex market dynamics more adeptly than traditional quant/cubist strategies.
Cammarano points out the diverse applications of AI in the realm of retail trading. He notes the technology’s utility in “quant/cubist short-term trading strategies, long-term market research, and short-term news/social sentiment analysis.”
Josh Kincaid, CMO at ToroAlerts, offers a critical perspective on the evolving role of artificial intelligence (AI) in retail trading.
He acknowledges AI’s profound impact, highlighting its advantages in speed, data analysis, and decision-making capabilities. However, Kincaid also raises concerns about potential overreliance on AI-driven strategies, suggesting that it could lead to a loss of critical thinking skills among traders and possibly exacerbate market volatility.
Kincaid provides compelling evidence of AI’s superior performance in trading through examples like The Medallion Fund and The C3 Fund, which have achieved remarkable returns by leveraging AI and machine learning. He points out, “Successful AI-based strategies…outperform traditional trading strategies in terms of performance and efficiency.” For instance, The C3 Fund’s staggering 876% return trading crypto in 2021 versus Bitcoin’s 24% illustrates AI’s potential to significantly outpace human trading strategies.
Kincaid also highlights real-world applications of AI for retail traders, from algorithmic trading to sentiment analysis and automated trading systems. He also notes the popularity of AI-driven strategies such as trading signals and copy trading among retail traders.
However, Kincaid also cautions about the mixed effects of AI trading bots and algorithms on market volatility and liquidity, including the potential for increased volatility through mechanisms like high-frequency trading and flash crashes.
Shawn Carpenter, Chairman and CEO of StockAlarm, draws compelling analogies and likens the advent of AI in trading to the transition from manual to self-driving cars.
Carpenter describes AI trading systems as “having a supercomputer sidekick that can crunch numbers, predict market moves, and even execute trades in the blink of an eye.”
Carpenter acknowledges the complexity of integrating AI into trading, stating, “jumping into AI trading isn’t as simple as downloading an app on your phone. It’s more like building a rocket in your backyard.” This analogy reflects the necessity of having a robust understanding of both financial and technological domains to effectively leverage AI in trading.
Exploring real-world applications, Carpenter envisions AI trading as possessing the ability to sift through “millions of tweets, news stories, and financial reports in seconds” for market sentiment analysis or to execute trades with astounding rapidity.
Highlighting AI’s role in risk management, Carpenter refers to it as a “guardian angel,” capable of forecasting market movements with remarkable accuracy and thereby facilitating better decision-making and portfolio management. However, he also points out the challenges AI introduces, including potential contributions to market volatility and liquidity fluctuations, describing the balance as “a delicate balance; we’re all still learning the ropes.”
Jonas Schleypen, the Co-founder & CEO of Hoc-trade Technology, highlighted how advancements in technology are making it easier for retail traders to leverage artificial intelligence.
Schleypen explains, “The barrier of technical knowledge and financial resources can be significant when leveraging AI for trading. However, especially in retail-focused AI applications, these barriers are getting lower.”
He points out that while some technical know-how remains necessary, the emergence of user-friendly AI applications is significantly lowering the entry barrier for retail traders. Schleypen illustrates this with an example, saying, “One may retrieve live sentiments from one provider, merge various other data sources based on the strategy, and have an initial code being written with ChatGPT.”
Regarding real-world applications, Schleypen mentions AI-driven strategies that are gaining popularity among retail traders, such as utilizing Natural Language Processing to scan for market sentiments in online forums. “Such sentiments, and especially strong sentiment changes, can be used as signals in trading strategies,” he notes.
On the topic of risk management and predictive analysis, Schleypen emphasizes AI’s crucial role. He introduces Hoc-trade, an AI-powered tool designed to monitor trading patterns, detect anomalies, and evaluate factors like consistency. “By providing timely alerts and insights, Hoc-trade empowers traders to make informed decisions and effectively manage their risk exposure,” he asserts.